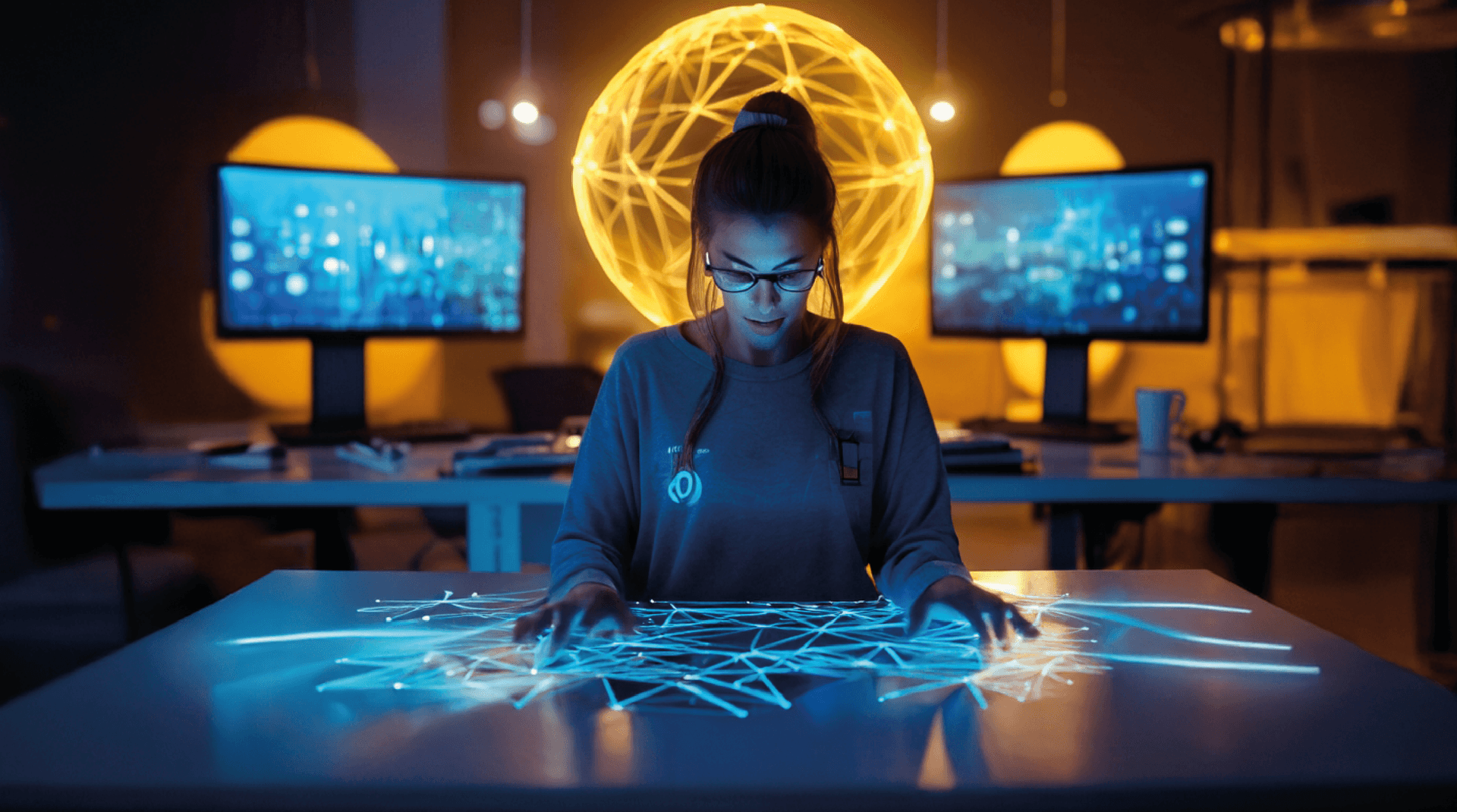
Reducing Downtime in Logistics with Custom Software Tools Tailored to Your Business Capacities
Speed and efficient logistics define a company’s competitiveness, yet many organizations still rely on legacy systems, putting themselves at increasing risk. Built on outdated technologies, these systems hinder adaptability and are ticking time bombs, set to become incompatible with modern digital platforms soon.
July 02, 20257 MIN